With more companies embedding AI in their commercial operations every day, AI is a rapidly growing market that has become an intrinsic part of our daily lives. But AI is also a digital disruptor that can inevitably increase risk, especially when it comes to handling and manipulating personal and sensitive data. Companies that use AI can address this risk by accepting their ethical responsibility to harness the technology in a trustworthy way.
But what exactly is Trustworthy AI (TAI), why does it matter, and whose responsibility is it? The unsettling answer is that if you’re a business user of AI, it is likely to be you. And if you’re an AI researcher, investor, or startup founder, it’s still important to understand what you should focus on or think about when it comes to the use of AI in practice.
What is TAI?
Trustworthy AI can be defined as business transformation with responsible AI solutions that address human needs, safety, and privacy. Specifically, TAI is defined by The Deloitte Trustworthy AI Framework as a methodology to help companies identify and mitigate potential risks related to AI ethics at every stage of the AI lifecycle. The framework includes the following categories:
- Transparent and explainable – clarity around how data is used and how decisions are made
- Fair and impartial – equitable application across all participants
- Robust and reliable – ability to learn from humans and other systems to produce consistency
- Safe and secure – protected from physical and digital harm
- Respectful of privacy – does not use data beyond its intended and stated use
- Responsible and accountable – governed by organizational structure and policies
Transparency and explainability are arguably the highest priority risks because they are key to being able to demonstrate each of the others in the framework.
Who is responsible for TAI?
The starting point for identifying who is accountable and responsible for AI is to look to the relevant regulation. Although AI has not been completely left out in the legal cold, aside from “guidance” or “proposals”, clear and specific established laws are lacking:
- There are older laws that could be reapplied to AI, such as SR 11-7 from 2011, which talks about model risk management specifically for banks.
- The 2016 GDPR mentions the right to explain automated decision-making, but not specifically AI.
- The U.S. FTC issued updated guidance in 2020 indicating that the use of an algorithm that resulted in discrimination would constitute “unfair or deceptive practices” prohibited by the FTC Act.
- Finally, there is softer regulation out of Europe, such as the UK ICO guidance 2020, as well as the EU AI Proposal 2021, which is not yet law but if passed could become the first ever legal framework on AI.
As a result of the lack of formal regulation, although the AI developer may have provided full service-level agreements and protocols to deploy the company’s new AI-powered silver bullet business tool, the fact is that it is the responsibility of the company that uses the AI to ensure that it is trustworthy.
What this means is that AI is a modern day global wild west, and the “era of self-regulation” is not over just yet. It is therefore vital that anybody involved in building or managing a company that leverages AI understands and takes ownership of their role as a self-regulator, which can be organized at the company, industry, or country level, for example:
- US Partnership on AI includes Microsoft, Amazon, Facebook, Apple, DeepMind, IBM and 100+ other partners (2016)
- OECD Principles on AI adopted a non-binding list of guidelines for the development and use of AI (2019)
- China AI Industry Alliance Guidelines for Self-Regulation (2019)
Operating in a new frontier environment can have huge benefits – it fosters exhilaration, creativity, and entrepreneurship. However, AI models are only going to become more complex as commercial AI adoption and regulation accelerates further. Companies must avoid building inherent risk into their business, and clear, robust, and transparent explainability is step number one.
Explainability as a core pillar of TAI
The Gartner AI Trust, Risk & Security Management (TRiSM) identifies explainability as a core pillar of Trustworthy AI. Explainability is a set of capabilities that describes a model, highlights its strengths and weaknesses, predicts its likely behavior, and identifies any potential biases.
It is often used interchangeably with interpretability, but it is important to recognize that they are not the same thing. Interpretability utilizes a mathematical understanding of numerical outputs of machine learning models. Most competitive interpretability mechanisms rely on graphical approaches that provide an added dimension to the quantifiable inputs and outputs.
However, a statistical understanding of the model is not enough. There needs to be an articulation of why weight was given to one input versus another, whether it was done automatically, and if a data scientist opened up the neural networks to change the weightings why this was done.
When and how big is the TAI opportunity?
Conversations regarding Explainable AI (xAI) date back decades, but the concept re-emerged in late 2019 when Google announced its new set of xAI tools for developers. In 2020, more vendors introduced improved explainable AI capabilities, and therefore, Explainable AI was less hyped compared to 2019, moving beyond the “Peak of Inflated Expectations” on Gartner’s 2020 Hype Cycle for Emerging Technologies. It has since reappeared on Gartner’s 2021 AI Hype Cycle as “AI Trism”, which is broader and now earlier in the cycle (5-10 years to plateau).
There are limited reports regarding the market size for Explainable AI since it is still so early. Some reports suggest an estimated market size of around $5 billion in 2021 with predictions that it will grow to $22 billion by 2030 with a CAGR of 20%. We believe the commercial market is actually likely to be much smaller today, but with immense prospects for growth, which is expected to come predominantly from industries that AI is likely to revolutionize – including banking, healthcare, manufacturing, insurance, digital government, smart transportation infrastructure, and autonomous vehicles. Regardless of industry focus, Gartner projected that 30% of government and large enterprise contracts will require XAI solutions by 2025.
Why is TAI so important?
A. Complexity
In order to be the most effective and accurate, AI models have become more sophisticated than ever before, which means they are also more difficult to understand than ever before. As per the image below, you can see the inherent tradeoff in AI between accuracy and interpretability as you move across the spectrum of model complexity.
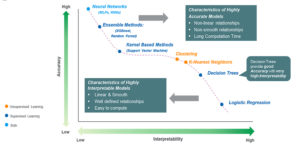
B. Bias and reputational risk
AI is only as good as the data it is trained on (as they say, garbage in, garbage out). Like a young child using training wheels to learn to ride a bike, maintaining direction and balance is vital to the effectiveness of an AI model. Explainability is one of the tools to detect and prevent bias, which is why it is essential that it is clear, robust, and understandable.
Combining poor explainability with weak underlying training data and a lack of monitoring capabilities can lead to disastrous negative consequences of bias emerging:
- Data-driven bias – When societal biases are demonstrated. One example from an MIT Media Lab study demonstrated that some major commercial facial analysis software contain inherent skin-type and gender biases, and can be up to 35 times more likely to misidentify darker-skinned women than lighter-skinned men.
- Proxy discrimination – When seemingly neutral factors act as proxies for other more biased classifiers. The Apple credit card did not have gender as a variable in its algorithm, but it still offered smaller lines of credit to women compared to men by using shopping records as a proxy for gender.
C. Consumer and corporate trust erosion
Even if there are no problems with the AI itself, an inability to simply explain the AI’s decisions can erode consumer trust in the products they’re using.
For instance, a good credit score applicant being rejected for a bank loan by an automated AI algorithm may not demonstrate something inherently wrong with the AI, but the highly sophisticated model has demonstrated a bias. The bank needs to be responsible for identifying and explaining in accessible terms exactly why the automated AI algorithm created that response.
However, in the absence of a single person accountable for AI, most customers in this scenario get a generic response from the bank to the effect of “Uh, I’m not really sure. The AI algorithms make all of our underwriting decisions,” which is just not an acceptable response to the customer. The risk management of AI must be a team sport involving collaboration from many different roles including IT, legal, compliance, operations, the front desk, etc., and everyone should be equipped to “explain” relevant AI-usage to customers.
Poor explainability can also erode the corporation’s own internal trust of systems and willingness to deploy AI. For example, the CEO or board may be thinking, “Even though this AI algorithm could improve our business performance or save us a lot of money, if we can’t trust the AI system we built, how can we actually use it to conduct business?”
D. Safety and security issues
The outcomes of bias or other faults within AI can vary. Some have specifically dangerous consequences, such as an inaccurate medical diagnosis for a cancer patient, or a car crash involving a self-driving vehicle. Others may bring reputational risk, particularly as AI becomes an integral component in building and maintaining brand reputation and customer satisfaction.
The time is now for those operating in the ‘TAI wild west’ to ensure they are not building inherent risk into their business. Companies need to be prepared for the galloping sophistication of models, commercial adoption, and regulation that lies ahead.
Next comes managing the risks around AI safety and security, which is a section in its own right. To be continued…
*Author’s note: If you have comments or would like to discuss TAI with us, please contact [email protected], as we’d love to hear from you!*